How Artificial Intelligence (AI) Is Transforming NVMe® SSDs
- Release Date: 2022-04-07 Browse Times: 141
Content
Artificial intelligence (AI) is sweeping across all industries and is transforming the world into a new technological era. Ever-growing AI applications encompass multiple fields such as automotive, data centers, cloud storage, healthcare, cyber security, speech/language recognition and more. AI owes this growth to its ability to analyze data efficiently and provide accurate results by using various algorithms.
Everyone experiences these powerful AI algorithms when opening a browser or social media platform and seeing a personalized product, dinner, or even travel recommendation that was based on prior browsing activity.
Storage is no exception. Methods to monitor data centers with fewer human interventions are needed to keep up with the scale of systems and technologies used. NVMe® Solid State Drives (SSDs) are keeping up with the demands for higher performance and data protection needs; however, they lack the technology for preventive measures for data loss and early detection of any functional degradation.
The adoption of AI and ML in NVMe SSDs is becoming a requirement for predictive analysis of fault and recovery mechanism, determination of traffic, optimization of performance, and for adaptive NAND management for improving reliability. Pervasive AI and ML across the entire datacenter infrastructure is now starting to include the endpoint SSDs.
Artificial Intelligence (AI), Machine Learning (ML) and Deep Learning (DL) are often used interchangeably, but they are all not the same. AI can be defined as building intelligent programs and machines that can creatively solve complex problems or mimic human thinking. Machine learning is a subset of Artificial Intelligence that provides systems the ability to automatically learn from the input training data and provide accurate output. Deep Learning is a subset of ML, which uses the neural networks to analyze varied factors with a structure that is like the human neural system.
To dive deeper, a Machine Learning model (ML engine) learns from past data, also called training data, to make predictions without explicit programming. When a new set of training data is introduced, the ML model trains again with the additional data to provide more accurate output data – as the amount of training data increases in the training data set, the accuracy of the ML output also increases. The most common and recognizable application of ML is auto-tagging suggestions on social sites. But there are also several other types of ML, such as:
- Supervised Learning – The learning algorithm takes the training (input) and the target labeled datasets, does the training (learning), and generates the accurate output
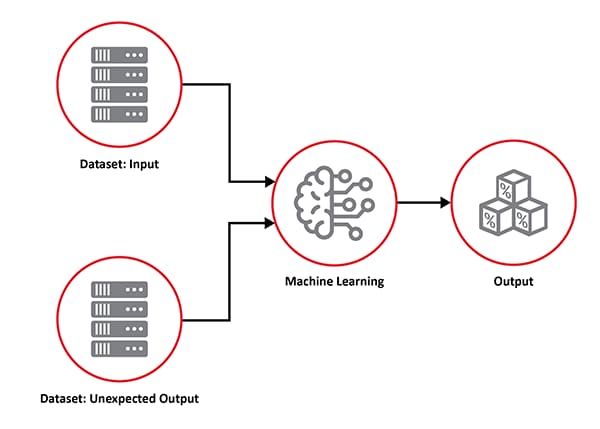
- Unsupervised Learning – The learning is done without the labeled data and the algorithm discovers patterns, data similarity or grouping in the input dataset
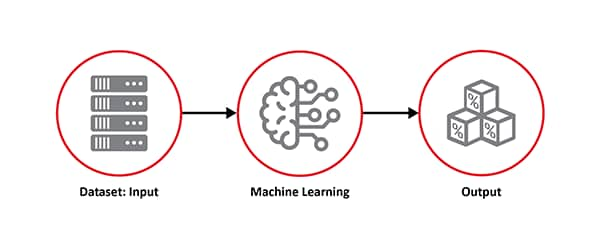
- Semi-supervised Learning - Combines supervised learning and unsupervised learning, allowing the algorithm to learn from a small amount of labelled content, while classifying a large amount of data
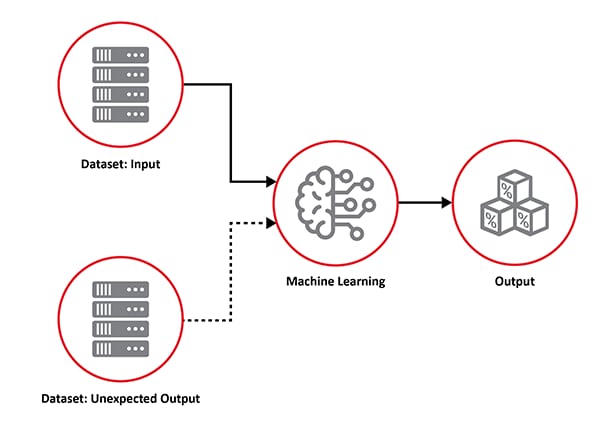
- Reinforcement Learning - Learning used to train over a period of time
How is an ML Model Created?
An ML model involves two modes of operation - a training mode and an application mode.
In the training mode, the ML engine has neural network nodes that need to be programmed with a functional model and then trained to learn and adapt. The training mode is done outside the NVMe controller.
The application mode is the runtime mode in the actual deployment stage, where the ML engine is continuously learning and adapting with the real environment and delivering the desired output. The ML engine configuration generated from the training mode is programmed into the NVMe Controller and used in mission mode.
How Does Having a Dedicated ML Engine Hardware Benefit the NVMe Controller?
A general-purpose ML engine can be designed in the NVMe controller for multiple applications such as:
Computational Storage: Turning the SSD into a Computational Storage Device (CSD) frees up computing resources and incorporates various AI and ML applications
SMART NAND Management: Learn varying behaviors of the NAND and choose the optimal set of parameters to be used with each NAND device in real time.
Improved Performance: Performance, endurance, and power can be improved with the use of ML engine by recognizing the IO request patterns, allocating and adjusting the data path resources, pre-fetching and keeping data ready in the local memory, using advanced cache management strategies, and applying dynamic power management strategies.
Quality of Service (QoS): Delivering consistent performance can be achieved by allocating and prioritizing the appropriate resources within the SSD.
Other Uses: Detecting and recovering from failovers caused by faults, firmware upgrades of security attacks, performing other self-tests and corrections, and scaling to various needs without detection.
Its important to remember when designing hardware implementations like an ML engine, it has to be generic enough to develop application agnostic AI and ML applications, but at the same time, it is important to balance performance, power, cost, and ease of use without compromising the data integrity.
Adoption of AI and ML hardware engines in the end point storage devices such as NVMe SSDs opens several creative applications for the data centers. It is a much-anticipated merger of two great technologies: AI and ML and NVMe SSDs. Microchip is excited to announce the availability of ML engine in their newest Flashtec PCIe Gen 5 NVMe 4016 SSD controller.
Read our white paper to learn more about the implementation of our Machine Learning engine or visit our website at microchip.com/Flashtec to learn more about the Flashtec PCIe Gen 5 NVMe SSD controller.